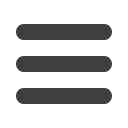
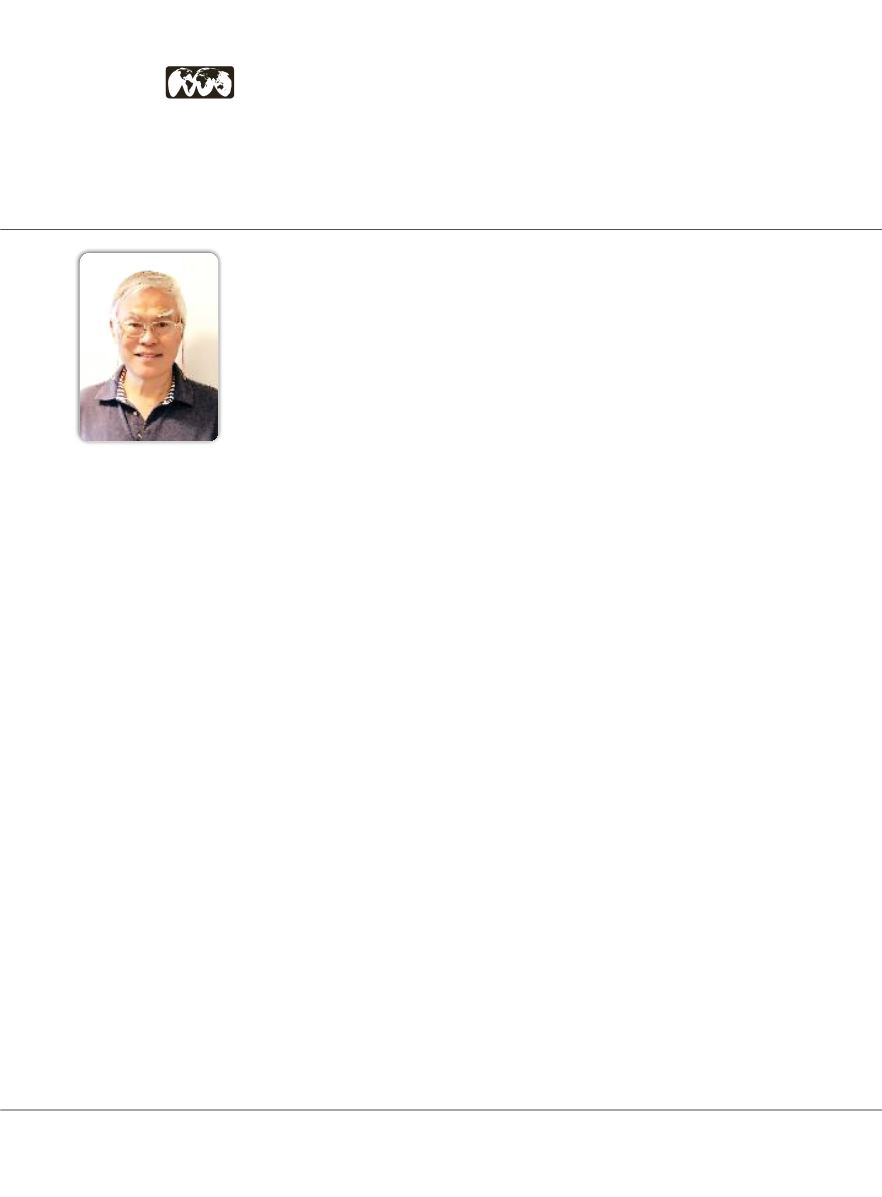
allied
academies
April 08-09, 2019 | Zurich, Switzerland
Health Care and Neuroscience
International Conference on
Page 16
Journal of Public Health Policy and Planning | Volume 3
Introduction:
This paper discusses type 2 diabetes
(T2D) patient’s glucose control guidelines from a
public health’s viewpoint. It is based on 1.5 million
data of chronic diseases and lifestyle details.
Furthermore, mathematics, physics, engineering
modeling, and computer science were used to
develop the needed models.
Method:
T2D is a serious worldwide epidemic
increasing at an alarming rate. Its complications,
especially cardiovascular disease (CVD) and stroke,
take many human lives each year. The author was
diagnosed with severe T2D 25 years ago and suffered
five cardiac episodes. He has spent more than 20,000
hours during the past 8.5 years to conduct a series
of research work on glucose control by using his
own developed math-physical medicine approach.
He believes in “prediction” and has developed five
models, including metabolism index, weight, fasting
plasma glucose (FPG), postprandial plasma glucose
(PPG) and hemoglobin A1C. All prediction models
have reached to 95% to 99% accuracy. His focus is on
preventive medicine, especially on diabetes control
via lifestyle management.
T2D patients have faced four major challenges:
(1) Awareness of disease and overcome “self-denial”
(attitude issue).
(2) Availability of correct disease information with
physical evidence or numerical proof (knowledge
issue).
(3) Determination and persistence on lifestyle change
(behavior psychology issues).
(4) A non-invasive, effective, and ease of use tool to
correctly predict glucose values (technology issue).
Results:
Let us put “psychological factors” aside for
the time being and just focus on practical methods
first. Any public health and healthcare professional
can apply the following techniques and tools to assist
T2Dpatients to put their glucose values under control.
Most of T2D patients can observe their improvement
on their glucose control within 90 to 180 days. Based
on meal quantity (including snacks and/or fruits) and
bowel movement, body weight can be estimated by
an APP tool, and therefore, FPG can also be predicted
consequently based on weight (FPG’s major factor).
The author developed this APP using optical physics,
wave theory, signal processing, energy theory, bigdata
analytics, and artificial intelligence (AI). It contains the
above mentioned five prediction models, including
the most sophisticated metabolism index model for
the overall health condition.
Gerald C Hsu
eclaireMD FoundaƟon, USA
From a public health’s viewpoint to address type 2 diabetes paƟent’s
glucose control issue (GH-Method: Math-Physical Medicine)