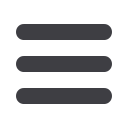
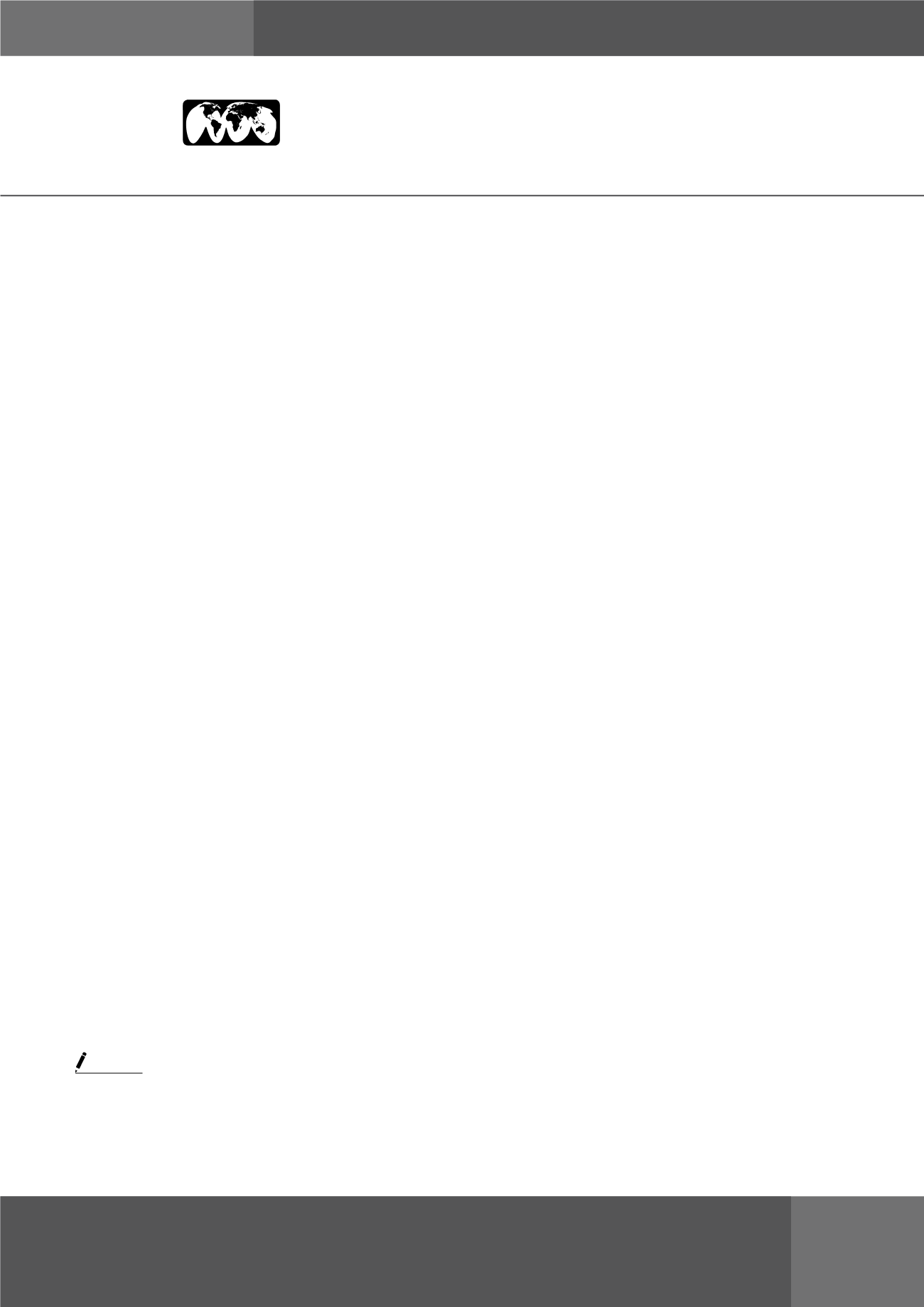
allied
academies
Journal of Biotechnology and Phytochemistry
Volume 1 Issue 3
Chemistry World 2017
Notes:
Page 54
November 13-15, 2017 Athens, Greece
7
th
World Congress on
Chemistry
Comparative evaluation of chemical mass balance
and multivariate receptor models using synthetic
data
Georgios Argyropoulos
and
C Samara
Aristotle University of Thessaloniki, Greece
I
n the field of atmospheric sciences, the term receptor models
is customarily used to describe top-down approaches for air-
pollution assessment, i.e. methods that begin by sampling air in
a given area, in order to match common chemical and physical
characteristics between source and air pollution samples. Source
identification and quantification is realized by employing
statistical analyses, widely known, under the term Multiple
Linear Regression (MLR). There are two fundamental categories
of RMs, Chemical Mass Balance (CMB) models, which assume
full knowledge of the compositions of emissions, andmultivariate
models, which apportion sources on the basis of observations
at the receptor site, alone. One of the first documented uses
of RMs for air-quality management was by the United States
Environmental Protection Agency (EPA), back in the early
80s. Since then, RMs have gradually become familiar to policy-
makers all over the world, as there were vast improvements, not
only in the MLR methods that can now be performed by modern
computers but also in the chemical speciation techniques that
provide RMs with input data. Nevertheless, there are still major
concerns regarding RMs, such as the influence of personal
judgment to model results, as well as the lack of a standard
methodology for quantifying uncertainty levels, since, in the real
world, one cannot check the model output, against the actual
values of source contributions. This study presents a comparative
evaluation of RMs, using synthetic input datasets, i.e. where the
values of source contributions are already known. Synthetic
data were generated by inducing random variations to reference
values, with the use of deterministic procedures, widely known
as “pseudo-random number generators”. Virtual receptors have
been set to match conditions that can actually hinder model
performance, such as large measurement errors, collinearity
between source profiles, strong correlations between the
temporal variations of source contributions etc. The simulation
includes the newest versions of CMB and multivariate receptor
models, as well as some of the previous ones that are still in
use, by the scientific community. Particular emphasis has been
placed on a recently developed computational procedure, the
so-called Robotic Chemical Mass Balance (RCMB), which has
been considered to be a mathematical optimization of previous
CMB models, minimizing personal judgment. Preliminary
results indeed confirm the superiority of RCMB over the human
modeler, if the latter one has under or overdetermined source
profile input data.
Biography
Georgios Argyropoulos is a postdoctoral researcher in the Department of Chemistry,
at the Aristotle University of Thessaloniki (AUTH), Greece. His educational
background includes an MSc degree in Chemical Engineering, an MSc degree in
Environmental Chemistry, and a PhD degree in Receptor Modeling, all received
from AUTH. One of his major research interests is the use of statistical techniques,
such as multivariate analysis, for source apportionment of atmospheric pollutants.
He has participated in numerous research projects, including the LIFE Environment
programme, funded by the European Commission. Recently, he also received a
Fellowship of Excellence for Postgraduate Studies in Greece, from the State
Scholarships Foundation (IKY) of Greece, in the framework of the Hellenic Republic
– Siemens Settlement Agreement.
geoarg@chem.auth.grGeorgios Argyropoulos et al., J Biotech and Phyto 2017