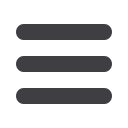
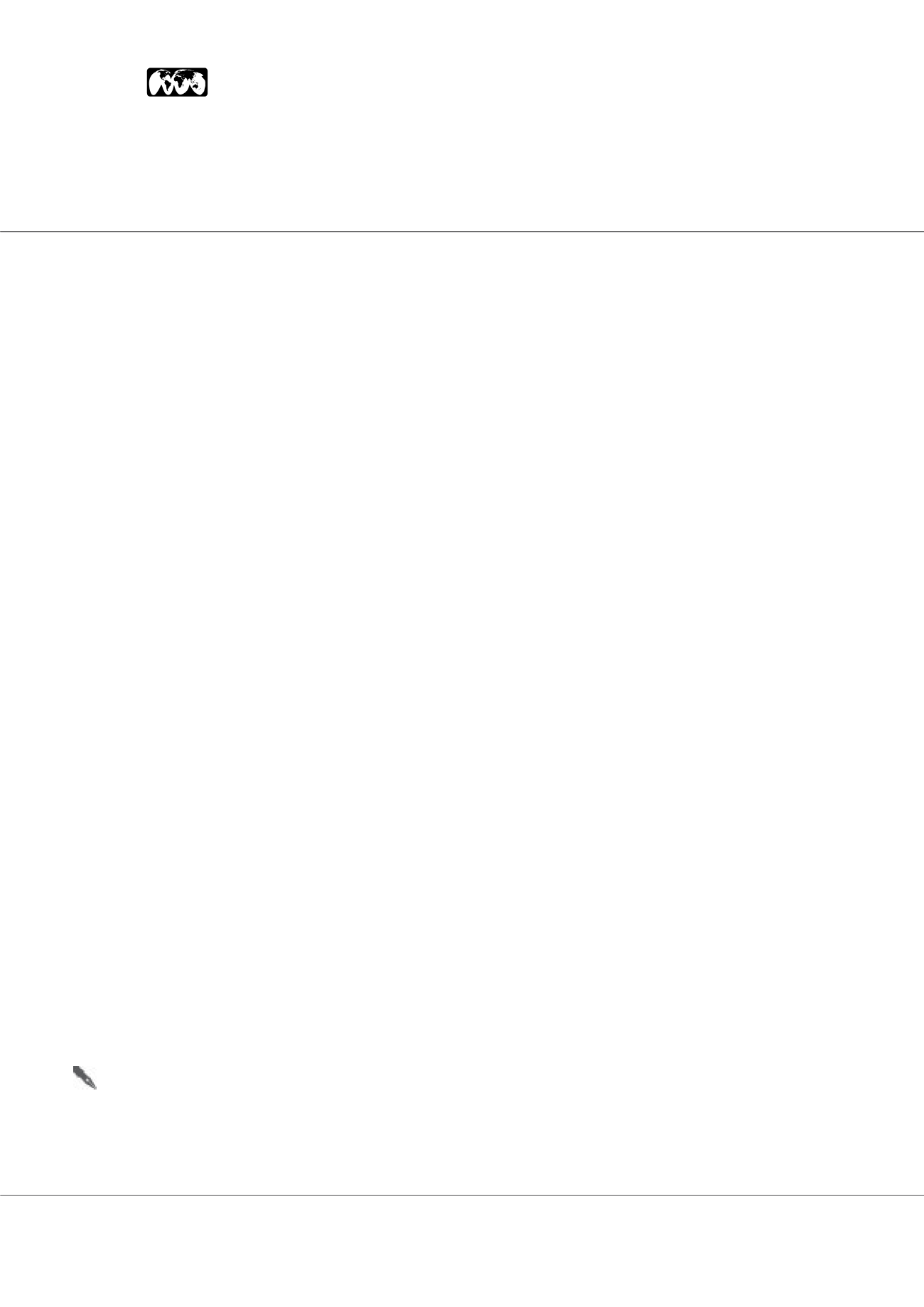
Page 48
Notes:
allied
academies
J Med Oncl Ther 2017 | Volume 2 Issue 4
Oncology and Biomarkers Summit
November 27-28, 2017 | Atlanta, USA
Annual Congress on
Heuristic multi-objective optimization algorithm to extract biomarker based on mutation combinations in
whole gene information for disease diagnosis
Yong-Joon Song
Korea Advanced Institute for Science and Technology, South Korea
T
here are still many efforts to diagnose diseases early.
Among them, molecular diagnostics using biomarker is
one of the powerful tools that can pre-diagnose diseases
before symptoms appear. In critical diseases such as acute
myeloid leukemia (AML), when the symptoms are present,
it is already late. It is possible to bring about complete
cure of cancer by performing preliminary examination and
early treatment based on molecular diagnostics. However,
researches related to biomarkers have been done only
from a biomedical point of view, focused on specific gene
sequences or protein expressions that are thought to be
related to disease. To overcome this stereotype, we proposed
an algorithm that uses a combination of disease-related
mutation information from entire gene. We used NGS data
of solid tissue normal samples from skin and primary solid
tumor samples from bone marrow, which were obtained
from 50 AML patients from TCGA database. In addition, we
extracted mutation information by using GATK tool. In order
to extract only cancer-related mutation information among
the obtained mutation information, we use a following
proposed algorithm. There are millions of mutations in the
entire gene, and a huge number of combinations. Thus, in
order to find biomarker with low complexity, we sorted all
the mutations by scoring how well the disease and normal
samples could be separated by each mutation. In this
process, the case of genetic mutation that occurs due to the
difference of skin and bone marrow was excluded. In the
derived list of mutations, we obtained optimal biomarkers
by heuristically solving three multi-objectives optimization
problems, which includes three parameters such as disease
classification ratio, the distance of inter-clusters and the
distance of intra-clusters. Using proposed method, we
could get a mutation combination that has 100% disease
classification performance for the sample we acquired.
Speaker Biography
Yong-Joon Song has completed his bachelor’s degree in Electrical Engineering at KAIST
in 2016. Currently, he is a PhD student in school of electrical engineering at KAIST. His
research interest area is Bioinformatics.
e:
yjsong@comis.kaist.ac.kr