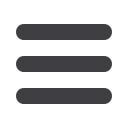
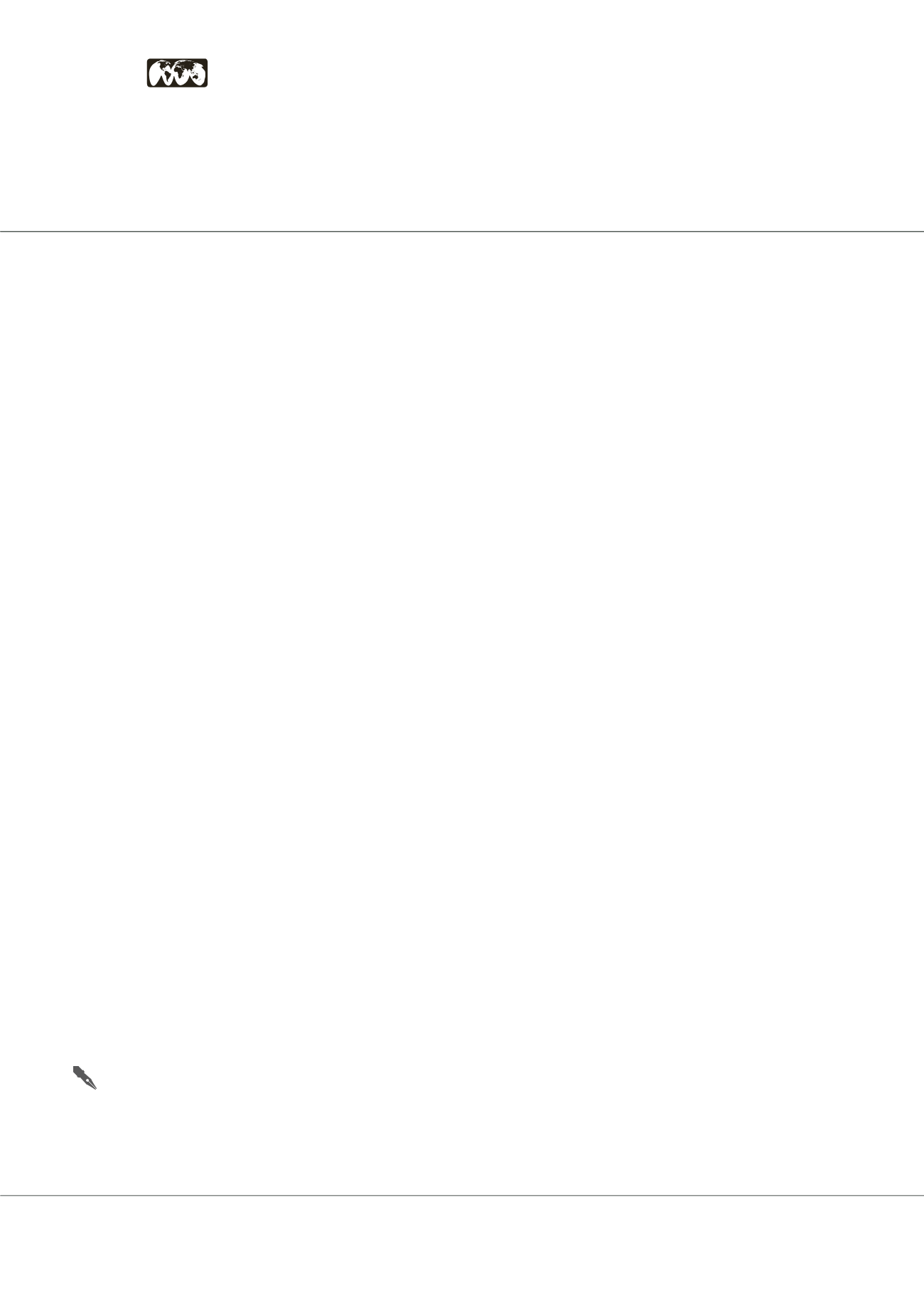
Page 30
Notes:
allied
academies
March 07-08, 2019 | London, UK
Journal of Diabetology | Volume 3
Annual Summit on
Diabetes, Obesity & Heart
Diabetes, Endocrinology and Metabolic Syndrome
International Conference on
Joint Event
&
Comparison of two clinic cases of using big data analytics and spatial analysis to investigate the
relationships among weight, glucose, blood pressure (GH-Method: Math-Physical Medicine)
Gerald C Hsu
EclaireMD Foundation, USA
T
he author uses math-physical medicine approach to
investigate three pairs of relationship between weight
vs blood pressure (BP), weight vs glucose (daily averaged
glucose, including both FPG and PPG) glucose and BP. There
are two clinic cases to be presented here. Case A uses lifestyle
management to control his metabolic disorders while Case B
uses both medications and partial lifestyle management to
control the same three chronic diseases. Both cases selected
the same time periods for results comparison: 1,770 days
(1/1/2014 - 11/6/2018) with big data of 17,700 metabolic
syndrome (weight, BP, glucose) each. This paper utilized two
statistical tools, i.e. time-series (x or y vs time, like EKG charts)
and spatial analysis (in a two-dimensional x and y space,
without “time” factor). In time-series graphs, if the correlation
coefficient (R) is greater than 50%, then these two sets of data
(or curves) are highly correlated to each other (i.e. strong). If
R is smaller than 30%, then considered as weak-correlated.
Since R can only be calculated for two sets of data (or curves),
therefore, this paper investigated 3 separate sets of pair
inter-relationships among these three metabolic elements. In
spatial analysis, if the “data cloud” is concentrated within a
long and narrow band and skewed with an angle (i.e. slope is
obvious and greater than zero), then these two sets of data
are highly correlated. On the other hand, if the angle of the
plotted point cloud is either flat or vertical, then there is a very
weak correlation between them.
Speaker Biography
Gerald C Hsu received an honorable PhD in mathematics and majored in engineering
at MIT. He attended different universities over 17 years and studied seven academic
disciplines. He has spent 20,000 hours in T2D research. First, he studied six metabolic
diseases and food nutrition during 2010-2013, then conducted research during 2014-2018.
His approach is “math-physics and quantitative medicine” based on mathematics, physics,
engineering modeling, signal processing, computer science, big data analytics, statistics,
machine learning, and AI. His main focus is on preventive medicine using prediction tools.
He believes that the better the prediction, the more control you have.
e:
g.hsu@eclairemd.com