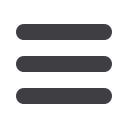
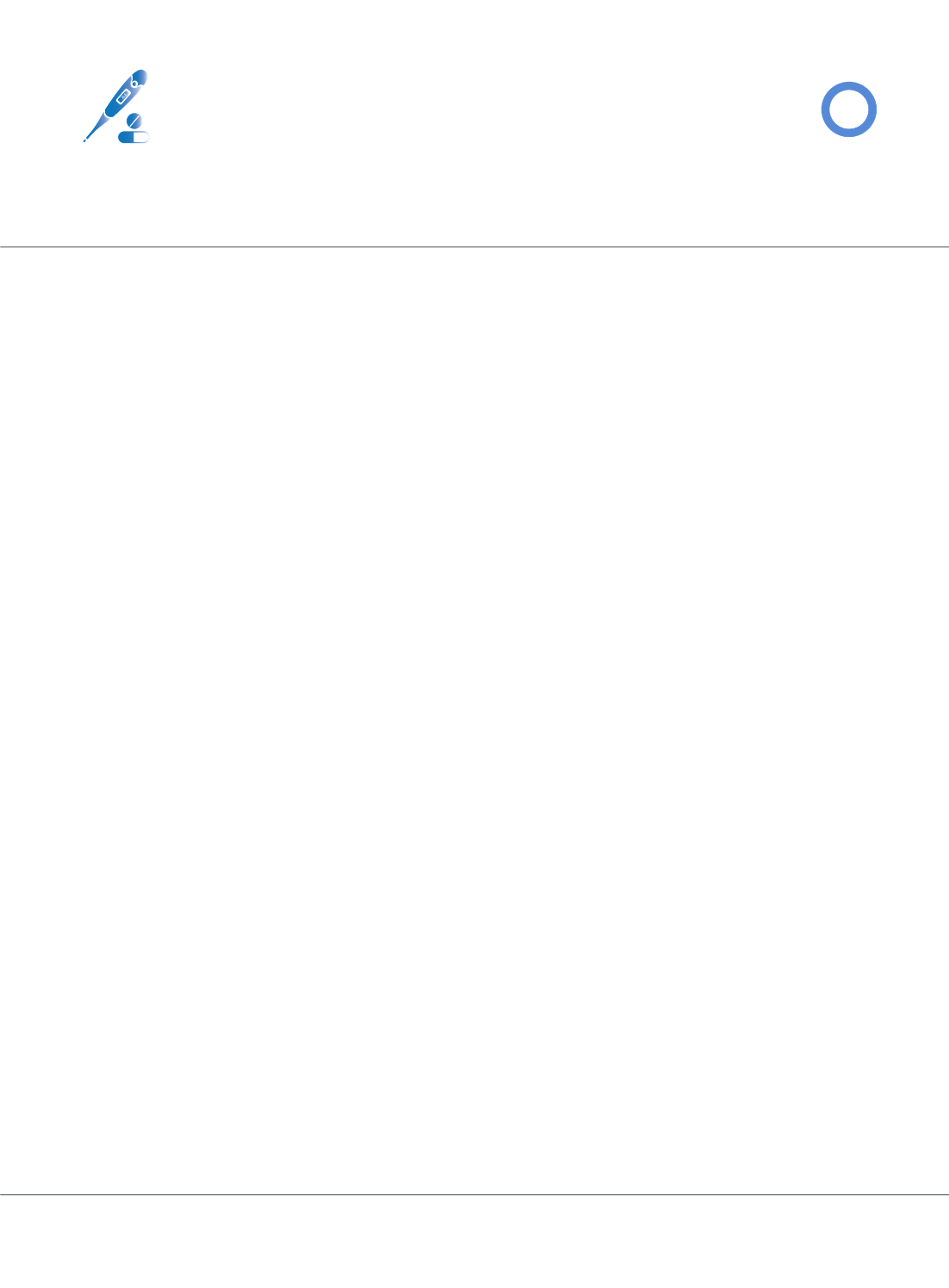
Page 46
Joint Event
November 29-30, 2019 | Frankfurt, Germany
28
th
International Conference on
3
rd
International Conference on
Diabetes and Endocrinology
Diabetes and Metabolism
&
2
0
1
9
CONGRESS
DIABETES
2019
DIABETES
Journal of Diabetology | Volume 3
Obesity biomarkers – Merging artificial intelligence with metabolomics
Flavia Luisa Dias-Audibert, Luiz C. Navarro, Diogo N de Oliveira, Jeany Delafiori, Carlos F O R Melo, Tatiane M
Guerreiro, Flávia T Rosa, Diego L Petenuci, Maria A E Watanabe, Licio A Velloso
and
Anderson R
University of Campinas, Brazil
O
besity––a condition characterized by body mass gain,
excess body fat, and risk for development of a number of
comorbidities––has become a worldwide epidemic affecting
more than 13% of the world population. One important
aspect affecting most obese subjects is the development
of a chronic, subclinical and systemic inflammation, one
of the contributing factors to the development of obesity
comorbidities. With advances in artificial intelligence,
researchers in the areas of therapeutic and diagnostic targets
are working to improve methodologies for more accurate
and sensitive identification of specific or set of biomarkers
able to predict risk for obesity-associated disorders, such as
type II diabetes. Within this context, we analyzed the plasma
of eutrophic and obese individuals by mass spectrometry
and performed data treatment using random forest-based
machine learning algorithms. Five biomarkers related to
inflammation in obesity were characterized: metabolites of
arachidonic acid, indicating the occurrence of inflammation;
molecules associated with dysfunctions in the nitric oxide
(NO) cycle and superoxide production; and a diabetes-related
species that may be the subject of future studies on the
trigger for diabetes in obesity. Calculated accuracy (90.8%)
and sensitivity (93.5%) for the model demonstrate that the
method is effective in separating groups as a function of
differential metabolite profiles given by mass spectrometry.
In other words, this work opens a new path for obesity in
metabolomics using advanced artificial intelligence strategies
for the election and determination of selective targets for
diagnostics, prognostics, and therapeutics.
e
:
flaviald.nutricao@gmail.com