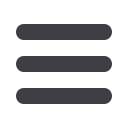
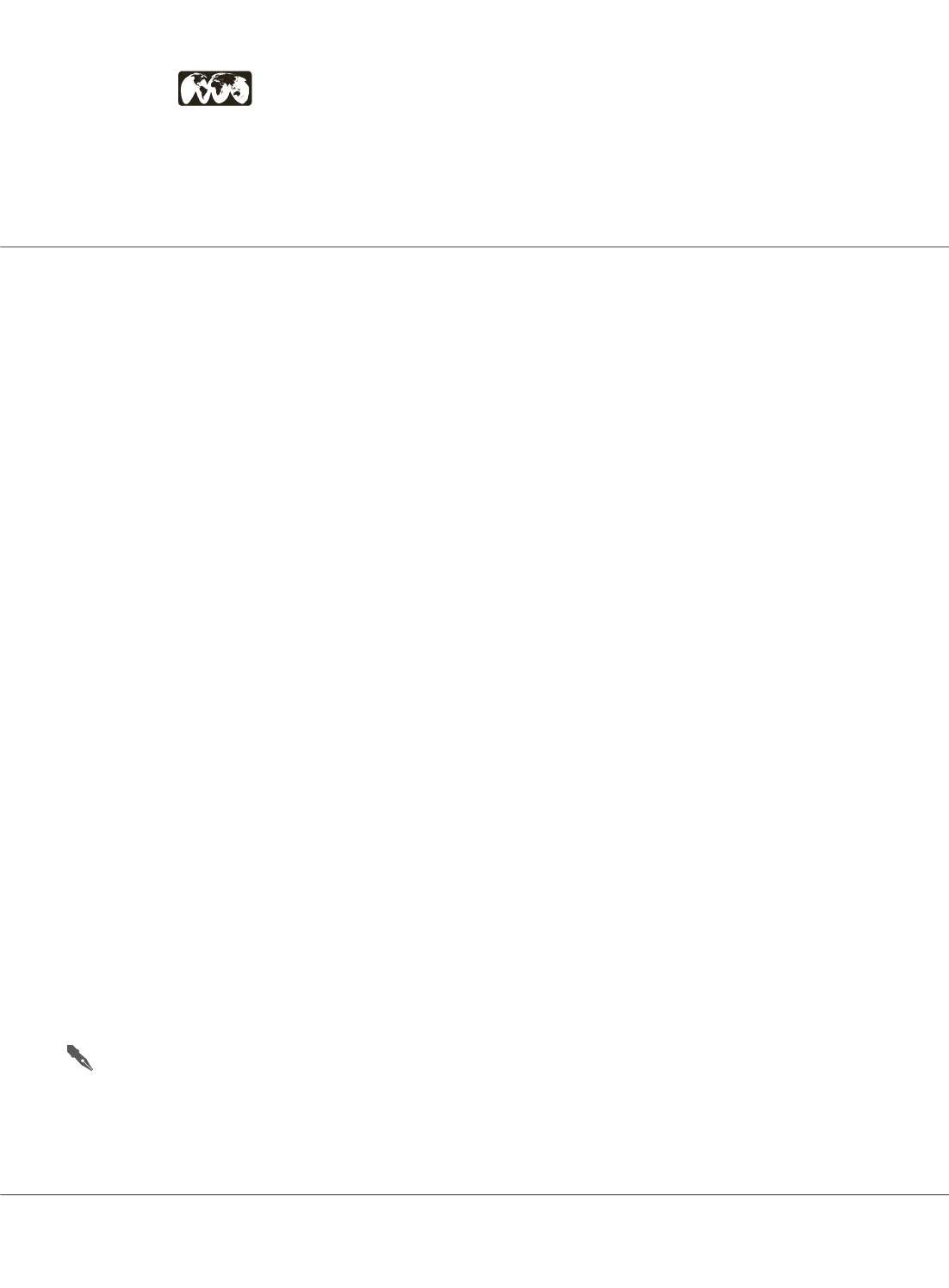
allied
academies
Page 42
Notes:
September 09-10, 2019 | Edinburgh, Scotland
2
nd
Global Summit on
3
rd
International Conference on
Dermatology and Cosmetology
Wound Care, Tissue Repair and Regenerative Medicine
Joint Event
&
Journal of Dermatology Research and Skin Care | Volume 3
Dermatol Res Skin Care, Volume 3
Machine learning analyses on data including essential oil chemical composition and in
vitro experimental antibiofilm activities from different bacterial belonging to either gram-
positive or gram-negative species
Rino Ragno
Sapienza University of Rome, Italy
M
icroorganisms and opportunistic pathogens can cause
persistent infections due to their peculiar antibiotic
resistance mechanisms and to their ability to adhere and
form biofilm. Biofilm resistance to antimicrobials is a complex
phenomenon, not only driven by genetic mutation inducing
resistance, but also by means of increased microbial cell
density that supports horizontal gene transfer across cells.
The interest in the development of new approaches for the
prevention and treatment of bacterial biofilm (BB) formation
has recently increased. Experimental data indicated that EOs
are able to modulate biofilm production of different Gram-
positive (
Pseudomonas aeruginosa
, PA) and Gram-negative
(
Staphylococcus aureus
and
Staphylococcus epidermidis
,
SA and SE) bacterial strains. In particular, EOs influenced
biofilm production with unpredictable results leading to
either BB inhibition or reduction depending both on EOs’
chemical composition and on type of microorganism. Aim
of this presentation is to demonstrate how application of
machine learning (ML) application to complex matrix of
data from 89 essential oils (EOs) chemical analysis and their
related in vitro experimental antibiofilm potencies can lead
to hypothesize on the mechanism of action of EOs’ chemical
components. To elucidate the obtained experimental
results, ML algorithms were applied leading to statistically
robust classification models. Analysis of the models in term
of feature importance and partial dependence plots led to
indicating those chemical components mainly responsible for
biofilm production, inhibition or stimulation for each studied
strain, respectively. Data from these investigations represent
the basis for future experiments that could enable to produce
blends of EOs specifically engineered to obtain more potent
anti-biofilmefficacy applicable inmany fields such as airborne
decontamination, products for dermatological and respiratory
tract infections, and others.
Speaker Biography
RinoRagno isanAssociateProfessorofMedicinalChemistryasDepartment
of Dug Chemistry and Technology of Sapienza University of Rome. He is the
coordinator of the Rome center for Molecular Design (RCMD) lab and has
published more than 120 papers in peer-reviewed journals in English with
more than 2700 citations
(scopus.comaccessed July 2019), an h-index of
31,3patents,fourbooksorbookchapters,presentedhisworkatnumerous
conferencesandsymposia.In2005hewasawardedbytheItalianChemistry
Society’s Medicinal Chemistry Division for his research in the medicinal
chemistry field. His main research fields are focused on the application
of computational methods to medicinal chemistry and extraction of
essential oils aimed to characterize them chemically and microbiologically.
e:
rino.ragno@uniroma.it