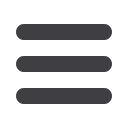
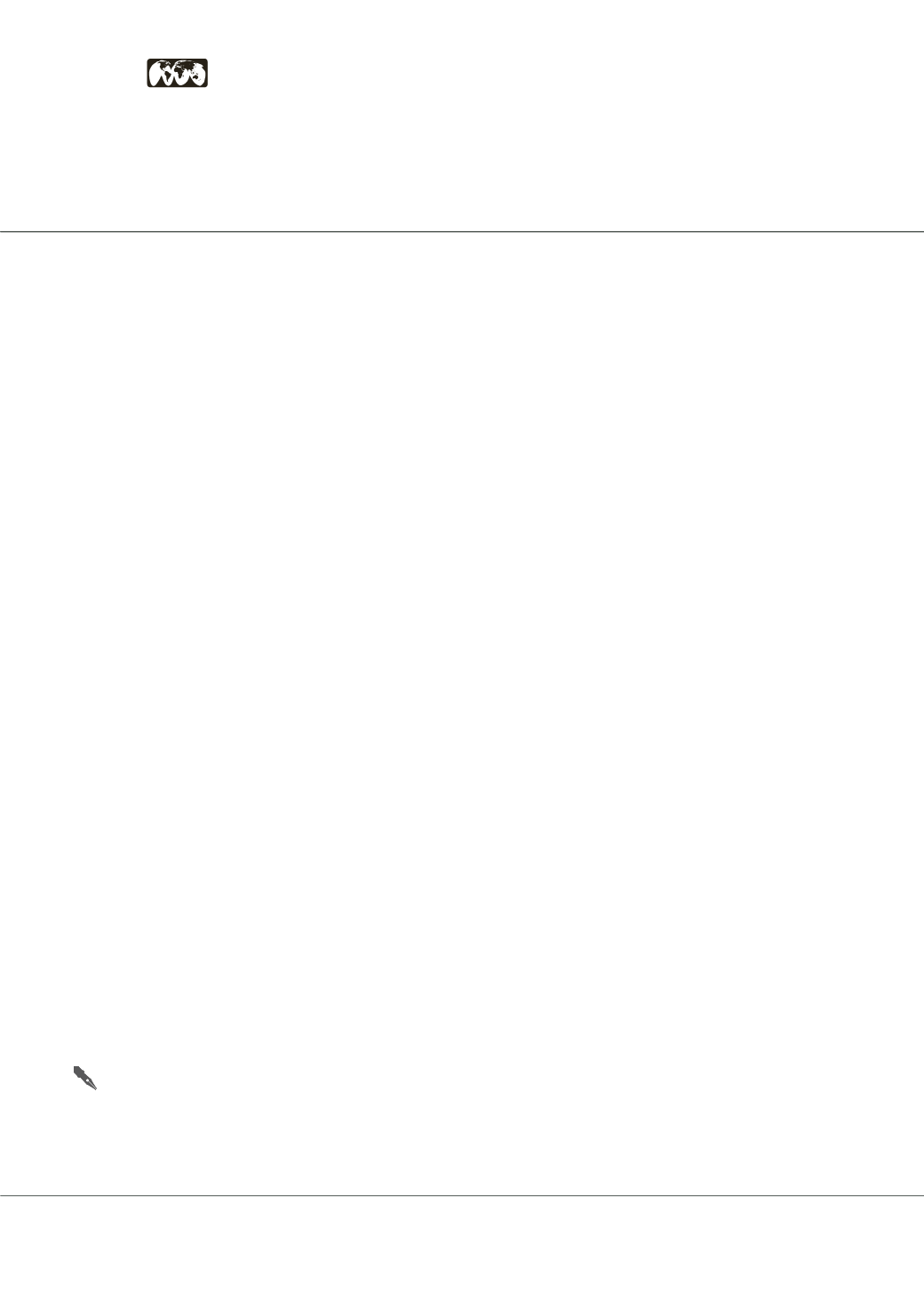
Page 31
Notes:
allied
academies
Nov12-13, 2018 | Paris, France
Central Nervous System & Therapeutics
International Conference on
Journal of Neurology and Neurorehabilitation Research | Volume 3
Automatic reporting of Lumbar Magnetic Resonance Imaging in patients with low back pain
Mohammed Al Jumaily
Dr. Sulaiman AL Habib Medical Group, UAE
Introduction:
Chronic Lower Back Pain (CLBP) is one of the
major types of pain that is affecting many people around the
world. It is estimated that 28.1% of US adults suffer from this
illness and 2.5 million of UK population experience this type of
pain every day. Currently the diagnostic imaging of the lower
back pain is mainly done by a visual observation and analysis
of the lumbar spine MRI images by radiologists and clinicians
and this process could take up much of their time and effort. In
addition, not all clinicians who see these images could interpret
them, these facts, therefore, rationalize the need for a new
approach to increase the efficiency and effectiveness of the
diagnostic imaging reporting.
Material and Methods:
We are proposing to develop a new
methodology to automatically aid clinicians in performing
diagnosis of CLBP. Our approach will be based on the current
accepted medical practice of manual inspection the MRI scans
of patients’ lumbar spine. The latter is done through visual
observation and analysis of specific slices in both axial and
sagittal views of the lumbar spine MRI. To detect lumbar spinal
stenosis and disc herniation, the clinicians locate the boundaries
of the different parts of the lumbar spine on the MRI image.
They then identify the distances between them.
Our proposed methodology will capture and model these
processes as algorithms. It starts with identifying slices in
a lumbar spine MRI that are useful and necessary for the
detection process. These slices are 2D at certain locations and
orientations. The images will be then divided spatially into
separate regions, each related to a specific organ by performing
image segmentation.
We developed a patch-based classification neural network
consisting of convolutional and fully connected layers to classify
and label pixels in the selected MRI slices. The classifier is
trained using overlapping patches of axial-view T2-weighted
MRI images of the bottom three intervertebral discs.
Results:
The results of the computer-aided MRI reporting
are highly sensitive and correlate very well with the human
radiologist reporting of the images.
Conclusion:
Computer-aided reporting of lumbar spine MRI
scans is a reliable method and could well reduce the cost and
time needed to report these images.
e:
maljunaily@yahoo.fr